Understand Statistics
Learn statistics in Hurry....
Ramnaresh
12/6/202433 min read
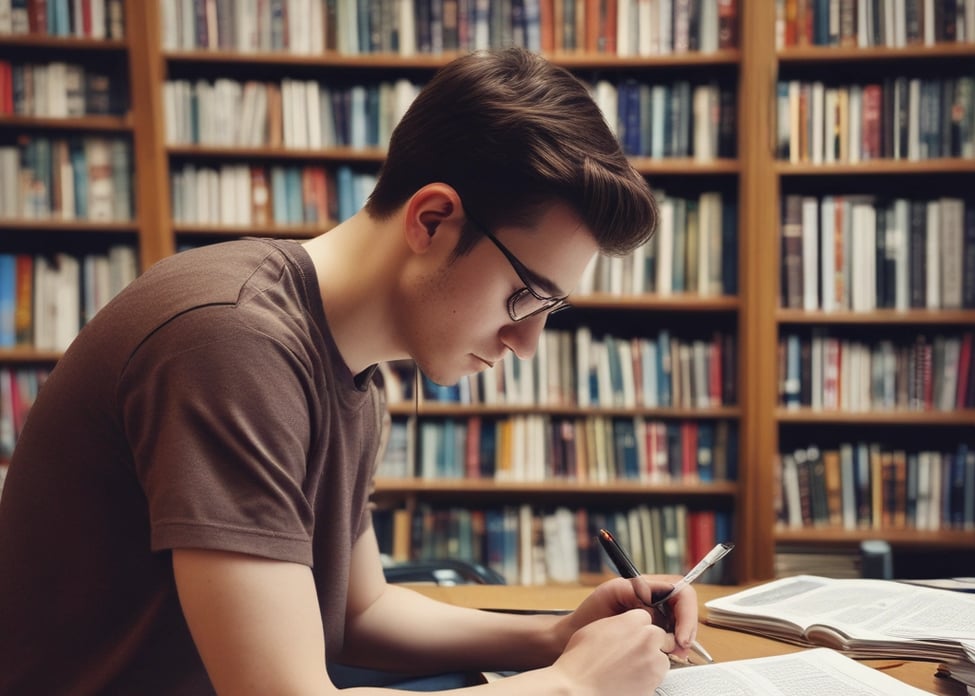
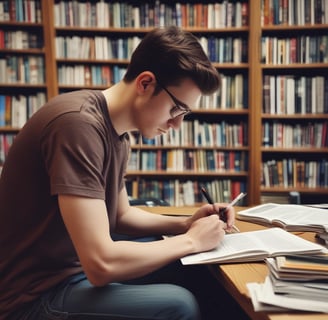
Learn Statistics in Hurry...
1. Descriptive Statistics & Data Visualization
Imagine you're in a room with several objects: a lamp, a chair, a table, a clock, and a bookshelf. Descriptive statistics is like organizing and describing these objects so that anyone who enters the room can understand what's there without having to look at every item closely. For example, the "average" number of objects in the room could be 5 (like the total number of objects divided by how many types of objects there are). A "spread" might be how far apart the lamp and the bookshelf are, while "central tendency" refers to where the majority of objects are placed, like towards the middle of the room. Data visualization is like creating a map or a picture of the room, showing how things are laid out. It helps someone visualize the distribution of objects at a glance.
2. Probability Theory & Applications
Think of a jar full of marbles, each a different color: red, blue, green, and yellow. Probability is like predicting the chance of pulling out a specific color marble. If there are 4 red marbles and 1 blue marble, the probability of picking a red marble is much higher than picking a blue one. The more marbles you have, the more accurate your predictions will be. So, in the universe of statistics, probability helps us anticipate outcomes in the world around us, like forecasting the weather or predicting a game’s score.
3. Sampling & Estimation
Imagine you’re in a massive library filled with thousands of books, but you only have time to pick a few to read. Sampling is like choosing a small section of books to represent the entire library. You pick some books from different shelves to get a general idea of what the whole library might offer. Estimation happens when you look at the few books you’ve chosen and guess how many more books in the library would be similar, based on what you’ve read.
4. Confidence Interval
Let’s say you're measuring the height of plants in a garden. You can't measure every single plant, so you measure a few. A confidence interval tells you that, based on those few measurements, the true average height of all the plants in the garden is likely to fall within a specific range. It's like saying, “I’m confident the average height of the plants is between 10 and 15 inches, with 95% certainty."
5. Hypothesis Testing
In a courtroom, a lawyer might make a claim (a hypothesis), like "The defendant didn’t cause the accident." Hypothesis testing is like the jury examining evidence to decide whether the claim is true or false. If the evidence doesn’t support the claim beyond a reasonable doubt, they reject it. In statistics, we collect data (evidence) to test whether a statement or assumption (hypothesis) is likely true or false.
6. Analysis of Variance (ANOVA)
Imagine you have three different types of cakes in front of you: chocolate, vanilla, and strawberry. You want to know if there's a difference in sweetness between the cakes. ANOVA helps you compare the sweetness of all three cakes at once, checking if the differences in sweetness are big enough to be considered significant, or if it’s just due to random chance. It's like asking, “Is there a real difference in taste, or are they all pretty much the same?”
7. Optimization & Linear Applied Algebra
Imagine you’re trying to find the shortest path to walk around the room while touching every object. Optimization is like finding that most efficient route. Linear applied algebra comes into play when you want to use mathematical equations to describe your steps and make sure they all lead to the shortest possible path. It’s like having a guide to help you navigate the room with the least amount of effort.
Each of these stories takes everyday objects and situations to explain statistical concepts, making them easier to understand and remember while keeping them engaging for your interviewer. This approach will not only demonstrate your knowledge of statistics but also your creativity in explaining complex ideas in a simple, relatable manner.
8. Correlation and Causation
Imagine you have a plant in your room and a window that lets sunlight in. As the sunlight increases, you notice that the plant grows taller. Correlation is like observing the relationship between sunlight and plant height — they seem to move together. But causation is like saying, "Sunlight is making the plant grow." Just because two things are related doesn’t mean one causes the other. For instance, the window might also allow fresh air in, and both sunlight and air might be contributing factors to the plant’s growth. So, correlation is observing the connection, while causation digs deeper to figure out which one truly causes the change.
9. Regression Analysis
Imagine you have a graph showing how the temperature outside changes as the time of day passes. Regression analysis is like drawing a line through all those temperature points to predict the temperature at any given hour. The line you draw represents the best guess, or prediction, based on past data. In real life, it’s like looking at a pattern in your room's lighting and using it to predict how much light you'll have in the future, based on time or season.
10. Normal Distribution
Picture a room full of people of different heights. If most people are around an average height and there are fewer extremely short or tall people, the distribution of heights forms a bell-shaped curve. The middle of the curve represents the average height, and as you move away from the center, fewer people fit those height ranges. The normal distribution shows that most things tend to cluster around a central value, like test scores, IQ, or the height of people in a population, with extreme values being rare.
11. Chi-Square Test
Think about two baskets: one filled with red balls and the other with blue balls. You have a hypothesis: the number of red and blue balls in both baskets is equal. The chi-square test is like checking whether the actual number of balls in each basket is significantly different from what you would expect. If the difference is big enough, you reject the idea that they’re equal, just like rejecting a claim in a courtroom when the evidence is strong enough to do so.
12. Time Series Analysis
Imagine you have a clock on the wall that ticks every second. Time series analysis looks at data that is collected over time — like observing the clock’s ticks. If you track the ticks over days, weeks, or months, you can see patterns: maybe the clock runs slightly faster or slower at certain times of day. Time series analysis helps us understand trends and predict what might happen next, based on past behavior. It’s like forecasting how many hours of daylight there will be tomorrow, based on trends from previous days.
13. Sampling Distribution
Now, imagine you have a giant jar of marbles, but you can only pull out a few at a time. If you keep drawing random samples of marbles and calculating their average color (say, average percentage of red marbles), you’ll get different averages each time. The sampling distribution is the collection of these averages. It shows how the averages of random samples are spread out, and it helps us understand what to expect if we’re only able to draw a few marbles, instead of looking at the whole jar.
14. Experimental Design
You want to test if a new type of chair is more comfortable than your old one. Experimental design is like setting up a fair test: you place people in the new chair and the old chair, making sure other factors like room temperature and time of day are the same. You want to isolate the effect of the chair alone. In an experiment, randomization helps ensure that you’re not accidentally introducing bias. It's like having an unbiased method for testing which chair will truly be the most comfortable.
15. Bayesian Inference
Imagine you’re in a room with several doors, and you’re trying to decide which door leads to a hidden treasure. At first, you might have no idea. But, as you gather clues (such as hearing noises or seeing hints), you revise your belief about which door is the best choice. Bayesian inference is like updating your beliefs based on new information. It allows you to constantly refine your predictions, adjusting them as you gather more evidence.
16. Central Limit Theorem
Picture you’re stacking dice, one on top of the other. Initially, each die is rolled randomly, and its result is unpredictable. But if you roll many dice and calculate the average of their rolls, the results start to form a bell-shaped curve. The central limit theorem tells us that, no matter how irregular the individual rolls are, the averages of large samples will tend to be normally distributed. It’s like saying, "If you keep adding more dice, the pattern of their averages will always look more and more like a normal distribution."
17. Statistical Significance
Imagine you're comparing two types of pens: one with blue ink and one with black ink. You test them by writing with each pen for 10 minutes and measure the amount of ink used. Statistical significance helps you determine whether the difference in ink usage is large enough to say it’s not just due to random chance. If the difference is statistically significant, you can confidently say that one pen uses more ink than the other.
18. Power of a Test
Think of trying to detect a faint sound in a noisy room. The power of a statistical test is like the ability of your ears to hear the sound over the noise. A more powerful test makes it easier to detect a real effect (like the sound). It’s important because if your test is weak (like your hearing), you might miss something important even if it’s there.
19. Multivariate Analysis
Imagine you're decorating a room with multiple elements: curtains, carpets, lights, and furniture. You might want to know how the color of the curtains affects the lighting or how the carpet texture influences the comfort of the furniture. Multivariate analysis is like looking at all these variables together to understand their combined effect on the room's overall aesthetic and comfort. It's not just about looking at each factor separately, but analyzing how they interact and contribute to the final result. This helps you make more informed decisions about the entire room’s design.
20. Outliers
In a room full of chairs, most of the chairs are of a standard height. However, one chair is unusually tall or short compared to the rest. This chair is an "outlier" — an observation that is much different from the others. Outliers are important in statistics because they can drastically change the results of your analysis. For example, if you’re calculating the average height of all the chairs, that one unusually tall chair could make the average look higher than it actually is. Identifying and understanding outliers helps to refine your conclusions and avoid misleading results.
21. Data Cleaning
Imagine you’re sorting through a pile of papers scattered across the room. Some papers are important, but others are crumpled, torn, or irrelevant. Data cleaning is like going through those papers and discarding the ones that are damaged or unimportant. You might also fix those that are slightly messy, like re-straightening them or filling in missing information. In data science, cleaning data ensures that the data you’re working with is accurate, consistent, and ready for analysis. Without proper data cleaning, your analysis could be flawed or incomplete.
22. Experimental vs. Observational Studies
Think of you conducting an experiment where you change the lighting in the room to see how it affects people’s productivity. This would be an experimental study because you’re actively manipulating a variable (lighting). On the other hand, an observational study is like watching how people in a different room work, without changing anything about their environment. You're observing what happens naturally. Both types of studies help you draw conclusions, but experimental studies give more control over the factors influencing the outcome, while observational studies rely on naturally occurring data.
23. Type I & Type II Errors
Imagine you’re conducting a test to see whether a new lamp can help people work faster. A Type I error would happen if you wrongly conclude that the lamp does help, when in fact it doesn’t (a false positive). A Type II error would occur if you fail to detect that the lamp does help, even though it actually does (a false negative). Both types of errors can affect the outcome of your experiment, and understanding them helps you design better tests to minimize mistakes.
24. Homoscedasticity and Heteroscedasticity
Consider the floor of your room. If the floor is level and even, it’s like homoscedasticity — the variance (or spread) of data remains consistent across different levels of an independent variable (like time of day). However, if the floor is uneven, with some areas higher or lower than others, it’s like heteroscedasticity — the variance of your data changes as the independent variable increases or decreases. In regression analysis, we assume homoscedasticity because uneven floors can make it harder to draw accurate conclusions.
25. The Law of Large Numbers
Imagine you’re flipping a coin. After just a few flips, you might get an uneven result (e.g., 3 heads and 1 tail). But the more times you flip the coin, the closer the results will get to 50% heads and 50% tails. The Law of Large Numbers explains this: as the number of trials increases, the average outcome tends to converge to the expected value. It's like saying, "With enough flips, the outcome will be predictable."
26. The P-value
Imagine you’re throwing a dart at a dartboard. The P-value is like asking, "How likely is it that I would hit the bullseye by chance?" A very small P-value means it's unlikely that you hit the bullseye by chance, so you can be more confident that you did it on purpose (i.e., your hypothesis is likely correct). A large P-value means the bullseye hit might just be a lucky accident, and you’re less confident in your hypothesis.
27. Clustering and Classification
Picture you're sorting a pile of mixed fruits into different baskets: apples, oranges, and bananas. Clustering is like grouping the fruits based on similarities, without knowing their exact categories ahead of time. You just see the natural clusters forming as you look at the fruits. Classification is like sorting the fruits into predefined categories, where you already know that an apple goes in the apple basket, an orange goes in the orange basket, and so on. Both are types of machine learning techniques used to group or categorize data.
28. Random Variables
Imagine a roulette wheel spinning in the middle of the room. The outcome of where the ball lands — on red or black, or on a number — is a random variable. It’s unpredictable and can take on different values, but it follows a certain distribution. The study of random variables allows statisticians to model uncertainty and make predictions about likely outcomes.
29. Markov Chains
Think of a game where you roll a die to determine where to move on a board. The rule is that your next move depends only on where you are currently, not on how you got there. This is like a Markov chain, where the future state depends only on the present state and not on the sequence of events that led to it. Markov chains are used in many areas, from predicting weather patterns to optimizing decision-making.
30. Network Analysis
Imagine a room filled with a group of people, each connected by strings. The strings represent relationships or interactions between people. Network analysis is like studying how these people are connected and how information or influence flows through the room. It’s a powerful tool for understanding complex systems like social networks, the internet, or even biological systems.
31. Confidence Level and Margin of Error
Imagine you’re aiming at a target with a bow and arrow. If you consistently hit around the center of the target, your aim is good, but there’s still a bit of variability — sometimes you hit a little to the left or right. Confidence level is like saying, “I’m 95% confident that my arrows will land within a certain distance from the center.” The margin of error represents how far from the center you can expect the arrows to land. A larger margin means more uncertainty, while a smaller margin suggests greater precision. In statistics, confidence levels and margins of error help us express how certain we are about the results of our data analysis.
32. Sequential Analysis
Imagine you’re baking cookies and testing them as they bake. Instead of waiting until all the cookies are done to check if they're perfect, you test a few cookies midway through the baking process. Sequential analysis is like this — instead of collecting all your data and then analyzing it, you continuously analyze data as it’s collected, adjusting your decisions in real time. This helps you stop early if you find clear patterns or decide to keep going if you need more data to be sure of your conclusions.
33. Simulation and Monte Carlo Methods
Let’s say you’re rolling a dice in different rooms of your house and counting how many times each number comes up. You don’t want to roll the dice a million times, but you want to understand the patterns. Simulation is like using a computer to model those dice rolls without actually rolling them, allowing you to quickly generate results. Monte Carlo methods are a special kind of simulation where you randomly sample possible outcomes to estimate the probability of something happening. It's like flipping virtual coins to understand the likelihood of various events occurring without physically carrying them out.
34. Bayesian Updating
Imagine you're trying to guess the contents of a closed box. Initially, you're unsure, so you make a wild guess based on the box’s size. But after shaking the box a few times and listening, you update your guess, maybe thinking it's more likely to be something fragile or soft. Bayesian updating is this process of revising your predictions as you receive new data. With every new clue, you adjust your initial belief about what's inside the box. In statistics, this concept allows you to continuously refine your estimates based on new information.
35. Sampling Bias
Imagine you’re trying to figure out what type of music people in your city like, but you only ask people at a concert. You’re missing out on the opinions of those who don't attend concerts. This is sampling bias — when your sample doesn’t represent the entire population because it's selected in a biased way. It's like trying to predict everyone’s music preferences based only on a group of people who already share the same interest, leading to skewed conclusions.
36. The Law of Total Probability
Let’s say you're organizing a scavenger hunt in your house, and there are three rooms where clues might be hidden: the kitchen, the living room, and the attic. You know the probability of finding a clue in each room, but you’re not sure where the clue will be. The law of total probability helps you calculate the overall probability of finding the clue by considering each room's likelihood and combining them. It’s like looking at all possible events and their chances to figure out the total chance of success.
37. The Concept of a Control Group
Imagine you’re testing a new type of plant fertilizer. You plant two sets of plants: one set gets the fertilizer, and the other set doesn’t. The control group is the set of plants that doesn’t receive the fertilizer. By comparing the growth of plants with and without the fertilizer, you can see whether the fertilizer made a real difference, as the control group serves as a baseline.
38. Factor Analysis
Picture a set of ingredients in your kitchen: flour, sugar, eggs, butter, and chocolate chips. Factor analysis is like grouping these ingredients into categories — dry ingredients, wet ingredients, and flavoring ingredients — based on their characteristics. It's a technique used to simplify complex data by grouping related variables. Instead of focusing on each individual ingredient, you analyze groups of them that work together, making it easier to understand the recipe.
39. Principal Component Analysis (PCA)
Imagine you have a large collection of photos spread out on a table. You want to understand the key features of the photos — the color, the lighting, the subjects — but it’s hard to analyze each photo individually. Principal Component Analysis (PCA) is like collapsing all those photos into a few main images that represent the most important features, reducing the complexity of the data without losing essential information. It helps you simplify large datasets by identifying the most important patterns in the data.
40. Dimensionality Reduction
Imagine a vast collection of books, each with hundreds of pages and chapters. Dimensionality reduction is like summarizing each book into just a few sentences that capture the main ideas. By reducing the number of dimensions (or details), you still keep the core information intact but in a much more manageable form. This technique is particularly useful when dealing with high-dimensional data where there are too many variables to process effectively.
41. Anomaly Detection
Let’s say you're in a room filled with candles, and most of them are lit. However, one candle is extinguished. Anomaly detection is like identifying this outlier candle — the one that behaves differently from the others. In data analysis, anomaly detection helps us identify unusual or unexpected patterns, like fraud detection in financial transactions or spotting faults in a machine.
42. Cross-validation
Imagine you're testing the strength of various materials for building a chair, but you can’t test every single piece of material available. Cross-validation is like taking a few different pieces from the pile, testing them in different ways, and checking how consistently the material performs across various tests. It’s a method used to ensure that your findings are reliable and not just based on one particular subset of data.
43. Hierarchical Clustering
Think of organizing a collection of books in your room into categories: fiction, non-fiction, science, history, etc. Hierarchical clustering is like grouping these books into a tree structure, where each main category branches out into subcategories (like fiction branches out into mystery, romance, etc.). This type of analysis allows you to see the relationships between items and how they fit into larger categories.
44. The Bootstrap Method
Imagine you want to estimate the average weight of apples in a basket, but you can't weigh every apple. The bootstrap method is like taking a small random sample of apples, measuring their weight, and then repeating the process many times, creating multiple smaller samples. By doing this, you can get a better estimate of the average weight of all apples in the basket, even though you're not measuring every single one.
45. Multi-Task Learning
Think of a person multitasking: they’re cooking, listening to music, and working on their computer at the same time. Multi-task learning is like training a model to solve multiple problems at once. Instead of focusing on one task, the model learns to recognize patterns and connections that help it address various tasks simultaneously. It’s a more efficient way to learn, just like multitasking can save time in real life.
46. Regularization (Lasso and Ridge)
Imagine you’re assembling a collection of books for a library, but you only have limited shelf space. You want to include the most valuable books without overcrowding the shelves with too many. Regularization is like adding a rule that limits the number of books you can select (Lasso) or limits how big or small the size of the books can be (Ridge). This helps you focus on the most important books, while avoiding clutter. In machine learning, regularization prevents overfitting by ensuring the model doesn’t get too complex or too specific to the training data.
47. K-Nearest Neighbors (KNN)
Imagine you’re trying to decide which movie to watch. You could ask your friends, but instead of asking everyone, you ask the people who have similar tastes to yours. K-Nearest Neighbors (KNN) is like finding the closest people who share your preferences and basing your decision on their past choices. The KNN algorithm works similarly by classifying data points based on their proximity to other data points. It helps make predictions based on the behavior of the "neighbors."
48. Time Series Analysis
Think of a clock on the wall that records the time every second. Time series analysis is like analyzing the pattern of the clock’s ticks over the course of a day or week, trying to understand how the time changes. In statistics, time series analysis involves looking at data points collected over time to identify trends, seasonal patterns, or irregular behaviors, helping you predict future values.
49. Sensitivity and Specificity
Imagine you’re trying to find a rare type of flower in a garden. Sensitivity is how good you are at finding that flower when it’s actually there — the higher your sensitivity, the more flowers you find. Specificity is how good you are at not mistakenly picking other flowers that aren’t the one you're looking for. Both sensitivity and specificity are key in evaluating the performance of a model, especially in classification tasks like medical testing, where you want to identify true positives and avoid false positives.
50. Hypothesis Testing and P-Value
Imagine you're trying to determine if a light bulb will last longer than another brand. You form a null hypothesis that both bulbs last the same amount of time. As you test the bulbs, the P-value tells you whether the differences in their lifetimes are statistically significant. A low P-value suggests the difference is real, and you may reject the null hypothesis (i.e., one bulb is superior). A high P-value suggests that the differences you observe could just be due to chance, and you fail to reject the null hypothesis.
51. A/B Testing
Think of you running a café and trying to decide between two types of coffee beans for brewing. You test both types by serving one type in the morning and the other in the afternoon to different groups of customers. A/B testing is like comparing two versions of something (like web pages or ads) to see which one performs better. You use the data from both tests to make an informed decision about which option is better.
52. Entropy and Information Gain
Imagine you're organizing a puzzle and trying to figure out the most efficient way to solve it. Entropy is a measure of how uncertain or mixed up the puzzle pieces are. When you make a move that reduces uncertainty (e.g., finding a piece that fits perfectly), you're gaining information. In decision trees, information gain is the reduction in entropy as you break down a complex decision into smaller, more manageable parts.
53. Bootstrapping
Imagine you have a large jar of jellybeans, but you don’t want to count every single one. Bootstrapping is like picking a few jellybeans randomly, repeating this process several times, and using the averages of those smaller samples to estimate the total number of jellybeans. In statistics, bootstrapping helps to estimate the accuracy of a sample statistic by creating many resampled datasets from the original data.
54. Gaussian Distribution (Normal Distribution)
Picture a pile of sand in the middle of a table, and you drop grains of sand from above. Most of the grains will fall near the center, creating a bell-shaped pile. Gaussian distribution, or normal distribution, is like this pile of sand, where most data points cluster around the mean, and the farther away you go, the less likely it is to find data points. This symmetrical bell curve represents how many natural phenomena (like heights, test scores, or errors in measurement) are distributed.
55. Linear Regression
Imagine you’re trying to predict how tall someone might be based on their age. You plot a series of data points on a graph, with age on the x-axis and height on the y-axis. Linear regression is like drawing the straight line that best fits through these points, representing the relationship between age and height. This line helps you make predictions about future heights based on age.
56. Chi-Square Test
Picture a large box filled with different colored marbles. You want to know if the distribution of colors is the same across several different boxes. The Chi-square test is like comparing the observed number of marbles in each color to the number you’d expect based on some hypothesis. If the difference is significant, you reject the hypothesis and conclude that the distribution is different from what you expected.
57. Monte Carlo Simulation
Imagine you’re trying to predict the outcome of a race between a few toy cars. Instead of running the race a hundred times, you simulate it using a computer to predict the likely winners. Monte Carlo simulations use random sampling to simulate different possible outcomes and are often used in situations where it’s too difficult or time-consuming to test all possibilities.
58. Poisson Distribution
Imagine you're monitoring how many cars pass by a street corner in a certain amount of time. If cars pass randomly but at an average rate, the number of cars you expect to pass during any time interval follows a Poisson distribution. This distribution helps model the occurrence of rare events over time, such as the number of accidents at an intersection or the arrival of customers at a store.
59. Central Limit Theorem
Imagine you have a bag full of marbles in different colors, and you randomly select a handful. Each handful might have a different mix of colors. However, as you keep taking more handfuls, the average distribution of colors will start to resemble a known pattern (like a bell curve). The Central Limit Theorem states that, no matter the shape of the original distribution, the distribution of sample means will tend to be normal if you take enough samples.
60. Confidence Interval
Imagine you’re trying to guess the weight of an object by measuring it repeatedly. The confidence interval is like saying, “I’m 95% sure that the actual weight of the object lies between this range.” It gives you a range of possible values where you expect the true value to be, based on your sample data.
61. Statistical Power
Imagine you're trying to find out if a light bulb lasts longer with a new energy-efficient coating. Statistical power is like the ability of your testing method to correctly detect whether the new coating actually makes a difference. If your power is high, you’re more likely to detect a difference if it exists. However, if the power is low, you might fail to notice a real improvement. In research, high statistical power reduces the risk of Type II errors (failing to detect a true effect).
62. Variance and Standard Deviation
Picture a group of kids measuring the height of plants in a garden. Most plants are roughly the same height, but some are taller or shorter. Variance measures how spread out those heights are from the average, while standard deviation is just the square root of variance, giving you a more intuitive measure of spread. It’s like checking how "far away" the plants are from the average height in a garden — a large variance means a bigger mix, and a smaller variance means most plants are similar in size.
63. Correlation and Causation
Imagine you notice that every time you eat ice cream, it seems to rain later in the day. Correlation means there’s a relationship between these two events, but that doesn't mean eating ice cream causes the rain. Causation is a stronger connection where one event directly affects the other. In statistics, it's crucial to remember that just because two things are correlated doesn't necessarily mean one causes the other.
64. Logistic Regression
Think of you organizing a game where you predict whether a ball will go into a hoop based on the angle and speed it’s thrown. Logistic regression helps you predict probabilities in situations where the outcome is binary — either the ball goes in the hoop (yes) or it doesn't (no). It’s like modeling the odds of success based on certain variables, helping you predict the likelihood of an event.
65. Z-Scores
Imagine you're comparing the test scores of two students. One scored 85, and the other scored 95. You want to know which score is better compared to the average performance of all students. A Z-score tells you how many standard deviations a score is away from the mean. A Z-score of 1.5 means the score is 1.5 standard deviations higher than the average, giving you a way to compare scores in a standardized manner.
66. Outliers
Picture a set of books lined up by size, but one book is much larger than the rest. This book is an outlier — an unusual data point that doesn’t fit with the rest of the data. Outliers can distort the analysis and can either be removed or handled with caution, depending on whether they represent an error or a unique observation.
67. Multivariate Analysis
Imagine you're analyzing the factors that affect a person's health: exercise, diet, sleep, and stress. Multivariate analysis is like looking at all these factors together rather than separately, seeing how they interact to affect overall health. It helps in understanding how multiple variables influence an outcome and provides a fuller picture of a situation.
68. T-Tests
Suppose you have two groups of people, one using a new app and the other using an old app. You want to know if the new app performs better. A T-test is a statistical tool that helps you compare the means of the two groups to see if the difference is statistically significant, helping you determine if the new app really is better or if the differences were just by chance.
69. Random Variables
Imagine you’re flipping a coin and recording whether it lands heads or tails. The outcome of each flip is a random variable because it’s uncertain, and each flip could result in either heads or tails. Random variables are fundamental in probability theory, as they represent the uncertain outcomes of experiments.
70. Markov Chains
Picture a person walking from room to room in a house, with a rule that they can only move to adjacent rooms. Their next move depends on where they are currently, but not on their past moves. This is like a Markov chain, where the future state (next room) depends only on the present state (current room) and not on the previous states. Markov chains model systems that undergo transitions from one state to another, with each step influenced only by the current state.
71. Skewness and Kurtosis
Imagine you're observing the height of plants in a garden. Skewness is like noticing whether the plants are more bunched up on the left side (negative skew) or the right side (positive skew) of the average height. Kurtosis, on the other hand, refers to how “tall” or “flat” the distribution is compared to a normal distribution. If the plants’ heights are very concentrated around the middle, it has high kurtosis, and if the heights are spread out more evenly, it has low kurtosis.
72. The Law of Large Numbers
Imagine you’re flipping a coin. The first few flips might give you more heads than tails, but as you keep flipping, the number of heads and tails will even out. The Law of Large Numbers states that as you collect more data (like more coin flips), the average results will converge toward the expected outcome (50% heads, 50% tails). This law ensures that with enough trials, randomness smooths out, leading to more reliable results.
73. Survival Analysis
Imagine you’re tracking the lifespans of light bulbs in your house. Some burn out quickly, while others last much longer. Survival analysis helps you estimate the time until an event occurs (like when a light bulb will burn out), taking into account the varying lifetimes of each bulb. It’s used in areas like medical research, where it helps predict the survival time of patients with different conditions.
74. AIC (Akaike Information Criterion)
Imagine you’re picking a movie to watch from a long list. Each movie has a score that tells you how good it is, but you also want to consider how long the movie is. AIC is like a score for a model that balances how well it fits the data (good score) with how simple it is (shorter movie). It helps you choose the model that provides the best trade-off between complexity and accuracy.
75. Entropy in Machine Learning
Think about sorting a box of mixed-colored balls into separate bins. If the balls are very mixed up, you have high entropy — high disorder. But if all the balls are sorted into bins by color, you have low entropy — low disorder. Entropy in machine learning is a measure of uncertainty or disorder, and it helps in decision-making processes, like building decision trees. The goal is to reduce entropy as much as possible, making decisions clearer and more predictable.
These analogies continue to break down complex statistical methods and concepts into simple, easy-to-understand scenarios. By illustrating the ideas with familiar examples, you make the material engaging and accessible, which will undoubtedly impress your interviewer with both your depth of knowledge and your ability to communicate that knowledge clearly and creatively.
76. Bayesian Inference
Imagine you’re trying to figure out whether it will rain tomorrow. You start with an initial guess based on your previous experiences — maybe you think there's a 50% chance of rain. Then, as you hear the weather forecast and observe clouds forming in the sky, you update your guess to 70%. Bayesian inference works the same way — you start with an initial belief (prior probability), and as new data comes in, you update that belief (posterior probability). It helps you revise your predictions in light of new evidence.
77. Principal Component Analysis (PCA)
Think of a large, complicated painting with many different colors. You want to describe it, but there are so many details that it's overwhelming. PCA is like zooming out and simplifying the painting into a few broad strokes that capture most of the essence of the image. In statistics, PCA helps reduce the complexity of high-dimensional data by transforming it into fewer dimensions, capturing the key patterns while ignoring the noise.
78. Multicollinearity
Imagine you’re trying to predict how well a plant will grow using the amount of water, sunlight, and soil quality as your predictors. If water and sunlight are highly related (for example, more water usually means more sunlight), then these two factors might confuse your model. Multicollinearity is when predictor variables are highly correlated, making it difficult for a model to distinguish which factor is truly affecting the outcome.
79. Mann-Whitney U Test
Suppose you're comparing the heights of two groups of people, but one group is taller on average than the other. You can’t assume the heights follow a normal distribution, but you still want to test if one group is generally taller than the other. The Mann-Whitney U test helps you compare two independent groups when their data isn’t normally distributed. It’s like comparing the position of two piles of sand to see which one is consistently higher.
80. Empirical Rule
Imagine a set of test scores where most of the students scored around 75, with a few scoring much higher or lower. According to the empirical rule, for a normal distribution, about 68% of the scores will fall within one standard deviation of the mean, 95% within two standard deviations, and 99.7% within three. It’s like knowing how most of the students’ scores are clustered and how far outliers are from the center.
81. K-Means Clustering
Think of a room full of people, each with a different level of enthusiasm. You’re asked to divide them into groups based on how excited they are. K-means clustering is like grouping them into clusters (like low, medium, and high enthusiasm), where each person belongs to the group with the closest average enthusiasm level. It helps organize data into distinct clusters based on similarity.
82. Shapiro-Wilk Test
Imagine you have a collection of measurements (like the height of people) and want to know if they follow a normal distribution. The Shapiro-Wilk test is a statistical test that helps you check if a sample comes from a normal distribution. It’s like checking whether the height of people is distributed in a bell-shaped curve or if there are some unusual patterns.
83. Confidence Level
Imagine you’re fishing in a pond and you catch a fish. Based on your experience, you say there’s a 95% chance that the pond has a lot of fish. Confidence level is like expressing how sure you are that your conclusion is correct. A 95% confidence level means you’re 95% sure that your estimate (like the fish count) is accurate based on the data.
84. Heteroscedasticity
Picture a bunch of bouncing balls. Early on, when they’re dropped, they bounce in a fairly consistent manner, but later on, as the balls hit the floor, their bounces get bigger and less predictable. Heteroscedasticity occurs when the variability of your data increases or decreases with the values of an independent variable. In regression, it means the error terms don’t have a constant variance, which can distort your model’s results.
85. Wilcoxon Signed-Rank Test
Imagine you’re testing whether a group of plants grows better after using a special fertilizer. You measure the growth before and after using the fertilizer. The Wilcoxon signed-rank test compares the differences in growth for each plant before and after, looking for any systematic improvement. It’s used when you have paired data and want to see if there’s a difference in the median.
86. Fisher’s Exact Test
Imagine you’re comparing the number of red and blue balls in two different baskets. You want to test if the distribution of colors is the same in both baskets, but the total numbers are small. Fisher's exact test is a statistical test that helps you determine whether the proportions in the two groups are different, particularly when sample sizes are small.
87. Poisson Regression
Suppose you’re counting the number of emails you receive each day, and you want to predict how the number of emails will change as you spend more time at work. Poisson regression is used when the outcome (in this case, the number of emails) is a count, and it models the relationship between the count and other variables (like time spent at work).
88. Causal Inference
Imagine you're trying to figure out whether eating a certain type of fruit makes people healthier. Causal inference is like setting up an experiment where one group eats the fruit and another doesn’t, then analyzing the data to see if the fruit really caused the health improvements. It’s about determining cause-and-effect relationships, rather than just observing correlations.
89. Exponential Distribution
Imagine you're waiting for a bus, and you know the average time between buses is 10 minutes. The exponential distribution models the time between events in a process where events happen continuously and independently, like waiting for the next bus. It helps estimate the likelihood that an event (like the next bus arriving) will happen within a certain time.
90. Survival Function
Think of a candle burning down. The survival function tells you the probability that the candle will last longer than a certain amount of time. In survival analysis, it represents the likelihood that a subject (like a patient or a machine) will survive past a certain time.
91. Box Plot
Imagine you have a set of numbers representing test scores, and you want to quickly understand the range and distribution of scores. A box plot is like drawing a box around the middle 50% of scores and using lines to show the minimum and maximum values. It helps you quickly see the spread of the data and identify outliers.
92. Time-to-Event Analysis
Imagine you're analyzing how long it takes for a plant to bloom after being planted. Time-to-event analysis is used to model the time it takes for a specific event to occur, such as the blooming of the plant, and it accounts for factors like the plant’s environment or care that might affect the timing.
93. Levene’s Test
Picture a group of people of different heights standing in a line. You want to test whether the variability in their heights is the same across different age groups. Levene's test is used to check if the variances between different groups are equal before performing further statistical analysis, like an ANOVA.
94. The Bootstrap Method
Imagine you’re building a model of a building using a set of toy blocks, but you’re not sure if your structure is stable. The bootstrap method is like randomly rearranging the blocks multiple times to get different views on how stable the structure could be. It helps you assess the variability and reliability of a statistic by resampling your data.
95. ROC Curve (Receiver Operating Characteristic Curve)
Imagine you’re trying to predict whether a ball will land in a basket, and you’re testing your prediction accuracy. The ROC curve helps you plot the trade-off between true positive and false positive rates, showing how well your model distinguishes between two classes. A good model has a curve that moves towards the top-left corner, indicating fewer mistakes.
These analogies keep adding to the richness of statistical concepts, using everyday scenarios to make advanced ideas accessible and engaging. This approach will not only demonstrate your understanding of statistics but also highlight your creativity in communicating complex ideas simply and effectively.
96. Sampling Distribution
Imagine you are testing the sweetness of apples by sampling a few from a large orchard. Each time you pick a different group of apples and measure their sweetness, you get slightly different results. Sampling distribution is like the distribution of these sample means (average sweetness) that you’d get from repeatedly picking apples at random from the orchard. It helps you understand how much variability you can expect when sampling from a population.
97. Chi-Square Test
Imagine you have a basket with red, blue, and green balls, and you expect them to be evenly distributed. After drawing some balls from the basket, you count how many of each color you got. The Chi-square test helps you compare your observed counts to what you expected, to see if there’s a significant difference. It's like asking, “Are the balls in the basket distributed in the way I thought they would be?”
98. Type I and Type II Errors
Think about a fire alarm system. A Type I error is like the alarm going off when there’s no fire — a false alarm. A Type II error is like the alarm failing to go off when there is a fire — missing a true event. In statistics, Type I errors mean detecting an effect when there isn't one (false positive), and Type II errors mean failing to detect a true effect (false negative).
100. Residuals
Picture a baker trying to make the perfect batch of cookies. The recipe says the cookies should spread evenly in the oven, but they turn out unevenly. Residuals are the differences between the predicted outcome (even cookies) and the actual outcome (uneven cookies). In statistics, residuals show how well the model fits the data — the smaller the residuals, the better the model.
101. Cross-Validation
Imagine you are testing a new recipe by trying it out on different groups of friends. Each time you test, you get feedback about the recipe's taste. Cross-validation is like testing your recipe on several different groups to see if it works consistently well across all of them. It helps in assessing how well your model will perform on unseen data.
103. The Central Limit Theorem
Imagine you’re sampling the heights of people in a large crowd. The Central Limit Theorem says that if you repeatedly take samples from the crowd and calculate the average height of each sample, the distribution of those averages will look like a normal (bell-shaped) curve, no matter what the distribution of individual heights is. It’s like making a batch of cookies from different ingredients — no matter how the ingredients differ, the batch’s final outcome is predictable.
104. Interaction Effects
Imagine you’re making a cake using two ingredients — flour and sugar. If you increase the amount of flour, the cake’s texture improves. But if you also increase the amount of sugar, the texture might worsen. Interaction effects occur when the effect of one variable (flour) depends on the level of another variable (sugar). In statistics, this concept helps us understand how variables work together to produce outcomes.
106. A/B Testing
Imagine you are running a bakery and you want to test two different types of chocolate chip cookies. You set up two tables: one with the traditional recipe and another with the new recipe. A/B testing is like comparing the results from two different versions (A and B) to determine which one is more popular or performs better. It’s often used in marketing and product development.
107. Logistic Function
Picture trying to predict if a seed will grow into a plant based on the amount of water it gets. A logistic function is like predicting the growth potential of the seed where the growth starts slowly, then accelerates, and eventually levels off as the plant reaches its maximum height. It’s used to model outcomes that have a limited range (like 0 to 1, where you’re predicting probabilities).
108. Non-Parametric Tests
Imagine you’re testing whether two sets of people prefer different flavors of ice cream, but the preferences don’t follow a normal distribution. Non-parametric tests are statistical tests that don’t assume any specific distribution of the data. They’re like comparing the preferences directly, without assuming that all preferences are spread in a certain way.
109. Gini Index
Imagine you’re organizing people in a room based on how much candy they have. If one person has all the candy and everyone else has none, the Gini index is high because there’s a lot of inequality. If everyone has about the same amount of candy, the Gini index is low. In statistics, the Gini index measures inequality in a distribution, commonly used in economics and decision trees.
110. F-Test
Think about comparing the variance (spread) in two different gardens. One garden has flowers growing in a very uniform way, while the other has flowers growing unevenly. The F-test helps you compare the variances of two groups (like garden types) to see if one has significantly more variability than the other. It’s used to test hypotheses about the ratio of variances in two samples.
111. Kaplan-Meier Curve
Imagine you're tracking the survival time of plants that were given different amounts of sunlight. A Kaplan-Meier curve is like a visual representation showing the proportion of plants that survive over time. It’s commonly used in survival analysis to estimate the probability of survival at each point in time, even with censored data (where some plants may not have been observed until the end).
112. Markov Decision Processes
Think about a robot in a maze trying to find the quickest path to an exit. At each point, the robot makes decisions based on its current position, and each action has a reward or cost. Markov Decision Processes are a mathematical framework for decision-making, where outcomes depend on current states, and the goal is to maximize long-term rewards.
113. Time Series Analysis
Imagine you’re tracking the temperature in a city every day for a year. Time series analysis helps you analyze patterns over time, such as trends, seasonality, and noise. It’s like predicting future weather based on past data and understanding how factors like time of year affect the temperatures.
114. Bayes' Theorem
Imagine you’re trying to predict the likelihood of a person getting sick, based on their symptoms. You start with an initial belief (prior probability) about how likely they are to be sick. As you observe more symptoms (new evidence), you update your belief. Bayes' Theorem helps you do this mathematically, combining prior knowledge and new evidence to make better predictions.